Researchers from Forschungszentrum Jülich and RWTH Aachen University in Germany have developed an open-source workflow for rooftop PV potential assessments from satellite imagery.
The new ETHOS.PASSION workflow can assess a given region's geographical, technical, and economic potential, as well as surface area, orientation, and the slopes of individual rooftop sections.
“ETHOS.PASSION also includes the detection of superstructures, i.e., obstacles such as windows or existing photovoltaic installations,” the scientists said. “The novel two-look approach combines two deep learning models identifying rooftops and sections, and an additional model for identifying superstructures.”
The workflow considers rooftop segmentation, section segmentation, and superstructure segmentation – all trained under U-Net architecture, which is an architecture that was first used for biomedical image segmentation and is now used for semantic segmentation. It relies on machine learning to separate images into meaningful parts.
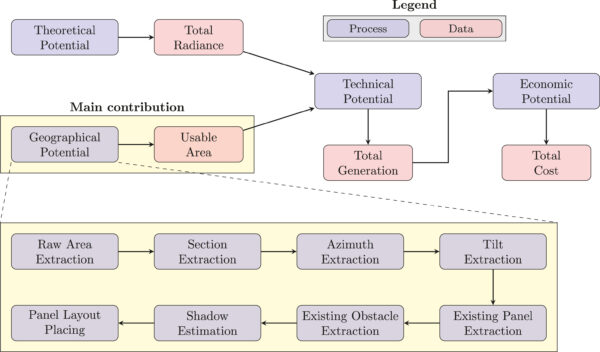
Image: Forschungszentrum Jülich GmbH, RWTH Aachen University, Solar Energy, CC BY 4.0 DEED
“Both the INRIA dataset for the rooftop segmentation and the RID dataset for the section and superstructure segmentations were split into 80%, 10%, and 10% for training, validation, and test sets,” the academics explained. After using some additional algorithms, the workflow's final output is the potential panel layout in the region. Based on this, technical and economic potentials can be further calculated.
The research group also used Intersection over Union (IoU), a common evaluation metric for image segmentation in computer vision. The IoU measures the percentage of overlap between the actual data and the prediction output.
In addition to introducing the novel workflow, the article proposed a conceptual frame of reference to compare different PV potential models. Their framework provides an implementation-independent point of reference that reduces ambiguity in academic information exchange and increases the comparability of methods, software, and data.
“The assessment of rooftop PV potential has become increasingly accurate due to the expanding availability of satellite imagery and improvements in computer vision methods. However, the analysis of satellite imagery is impeded by a lack of transparency, reproducibility, and standardized description of the methods employed,” the academics said.
They presented the new tool in “ETHOS.PASSION: An open-source workflow for rooftop photovoltaic potential assessments from satellite imagery,” which was recently published in Solar Energy.
This content is protected by copyright and may not be reused. If you want to cooperate with us and would like to reuse some of our content, please contact: editors@pv-magazine.com.
By submitting this form you agree to pv magazine using your data for the purposes of publishing your comment.
Your personal data will only be disclosed or otherwise transmitted to third parties for the purposes of spam filtering or if this is necessary for technical maintenance of the website. Any other transfer to third parties will not take place unless this is justified on the basis of applicable data protection regulations or if pv magazine is legally obliged to do so.
You may revoke this consent at any time with effect for the future, in which case your personal data will be deleted immediately. Otherwise, your data will be deleted if pv magazine has processed your request or the purpose of data storage is fulfilled.
Further information on data privacy can be found in our Data Protection Policy.