An international group of scientists has developed a novel algorithm for optimizing energy trading within cooperative renewable energy microgrids. The method utilizes particle swarm optimization (PSO) and gravitational search algorithms (GSA) with Nash Bargaining.
“Inspired by social behavior, PSO excels in local search and refinement of solutions, while GSA, inspired by the law of gravity, emphasizes global exploration,” the researchers explained. “The combination of these algorithms aims to strike a balance between exploration and exploitation, enabling efficient and robust energy trading strategies.”
The group calibrated some parameters of this hybrid model, following preliminary trials. Then, they added the Generalized Nash Bargaining (GNB) technique, which helps balance the interests of the different microgrids and facilitates fair energy trading outcomes.
After its development, the novel algorithm was implemented into the MATLAB software, where it was asked to solve a trading problem of energy cost minimization. This simulation was conducted with four interconnected microgrids with distinct generation sources: one with only PV, one with only wind power, one with both renewable energy sources, and one with none. All these system configurations were tested under varying energy prices and intricate load profiles.
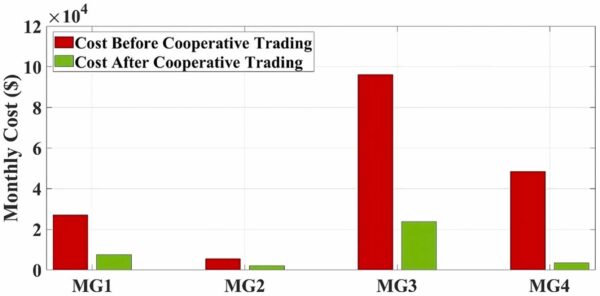
Image: Huazhong University of Science and Technology, Alexandria Engineering Journal, Creative Commons License CC BY 4.0
In addition, the optimization solution for the microgrid trading was compared to a base scenario. Each of those four microgrids could only trade with the utility grid in the base scenario.
According to the results, the overall monthly cost for all four microgrids was $94,551 without energy trading. However, utilizing the PSO-GSA model with Nash Bargaining in a scenario where the microgrids can trade with each other has reduced their total bill to $60,720.
Popular content
“It can be argued that the energy cost has sufficiently been reduced due to the implementation of the introduced cooperative energy trading within the microgrids,” the scientists said. “For example, in the base case, microgrid 1 has to pay the utility grid bill amount of $749.10 (for 24 hours) for the purchased energy from the main utility grid; however, after the cooperative trading, the same bill amount has been reduced to $500.25.”
In addition, the academic group has compared the novel optimization with four other metaheuristic algorithms under the same data and conditions. While the novel PSO-GSA has an energy cost of $60,720, GWO (Grey Wolf Optimizer) achieved $66,582. MPA (Marine Predator Algorithm) got $64,166; PSO alone got $60,925; ETSO (Enhanced Transient Search Optimization) achieved $61,122; and GSA alone yielded $60,994.
“The proposed hybrid PSO-GSA algorithm outperforms other optimization approaches in terms of its convergence characteristics and its capability to minimize energy costs,” the academics concluded.
The results of the simulations were presented in “Optimal energy trading in cooperative microgrids considering hybrid renewable energy systems,” published in the Alexandria Engineering Journal. The research team included scientists from China's Huazhong University of Science and Technology, Pakistan's COMSATS University Islamabad, Saudi Arabia's Taibah University, and Egypt's Ain Shams University and Future University.
This content is protected by copyright and may not be reused. If you want to cooperate with us and would like to reuse some of our content, please contact: editors@pv-magazine.com.
3 comments
By submitting this form you agree to pv magazine using your data for the purposes of publishing your comment.
Your personal data will only be disclosed or otherwise transmitted to third parties for the purposes of spam filtering or if this is necessary for technical maintenance of the website. Any other transfer to third parties will not take place unless this is justified on the basis of applicable data protection regulations or if pv magazine is legally obliged to do so.
You may revoke this consent at any time with effect for the future, in which case your personal data will be deleted immediately. Otherwise, your data will be deleted if pv magazine has processed your request or the purpose of data storage is fulfilled.
Further information on data privacy can be found in our Data Protection Policy.